What we know, what we miss and why TB persists
In a lab in the heart of Boston, professor Bree Aldridge leads a team of researchers trying to understand a threat that is both microscopic in size and global in consequence. The subject of Aldridge’s research is the bacterium Mycobacterium tuberculosis, the causative agent of tuberculosis. In 2023, TB made 10.8 million people sick and killed around 1.25 million people.
Mycobacterium tuberculosis has been infecting hominids even before the emergence of Homo sapiens over 200,000 years ago. Scientists have known about this bacterium and its role in causing disease for almost 150 years. However, despite the bacterium’s long-standing existence and the scale of devastation it causes, “We still don’t know a lot about the basics of TB,” Aldridge explained.
“The most fundamental things you can ask of a bacterium: How does it grow and divide? How does it control its cell cycle? Those are things that we don’t understand for this pathogen,” Aldridge said. “That’s why I started working on TB.”
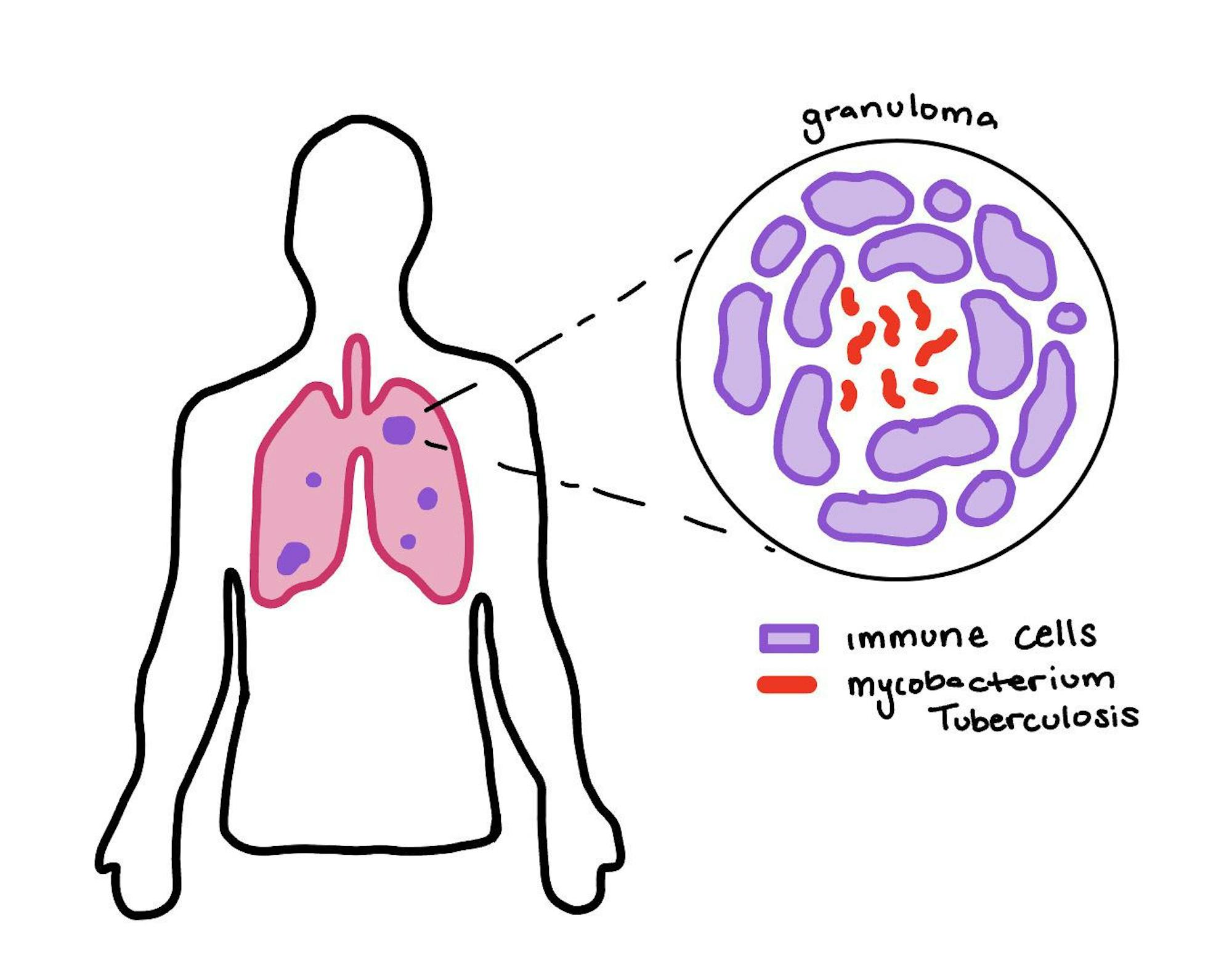
An illustration of granuloma in the lungs is pictured.
A term that comes up over and over again in Aldridge’s research is “heterogeneity.” Heterogeneity describes differences within a population. Aldridge wants to know the differences between bacterial cells in the context of a TB infection. How do these differences influence both drug susceptibility and the bacteria’s ability to persist within the human body?
When someone is infected with TB, their immune cells swarm to encircle it in an attempt to contain the infection. This creates “caves” that trap Mycobacterium tuberculosis, known as granulomas.
“[Granulomas] change over time, but they’re independent. So the environment that the bacteria are in in one granuloma [is] going to be different from what they are in a different granuloma, which means that they’ve adapted to different growth conditions and that gives rise to different susceptibilities,” Aldridge said.
Heterogeneity can be found within a single granuloma, and there can also be differences between granulomas themselves. These nuances in heterogeneity were explored in a recent paper from the Aldridge Lab published in Nature Microbiology in November 2024.
The common understanding of bacterial growth patterns comes from model organisms such as Escherichia coli. E. coli replicate by adding cell wall material in the center of the cell, and then they divide into two identical daughter cells. However, work from the Bree Aldridge Lab recently revealed that Mycobacterium tuberculosis replication does not fit this model.
Mycobacteria grow from their poles, meaning that material is added at either end of the cell rather than in the middle. Moreover, the Aldridge Lab used time-lapse, single-cell imaging to discover that the two poles of Mycobacterium tuberculosis grow at different rates. This means that one end will end up growing faster than the other, so when the cell divides, the two resulting cells have different properties and behavioral patterns. This is likely one of the reasons that while most bacteria grow at an exponential rate, Mycobacterium tuberculosis grows at a linear rate instead.
Computational biology and combination therapies
While pursuing her doctorate in biological engineering, Aldridge was working in the field of computational cancer biology.
“I was a quantitative cell biologist, and I was really struck that a lot of those impactful technologies and approaches weren’t being applied as much to infectious diseases — that all the new fancy tools were being used in cancer biology. And I wondered why we’re not bringing that same sort of innovative approaches to infectious diseases,” Aldridge said.
Following the completion of her doctorate, she made the switch to TB research. She still brings her computational background into her current lab’s other focus: optimizing drug combinations for TB treatment.
Because of the vast diversity of TB cells that can exist within one patient, drug combinations are needed to effectively treat the infections. There are three major ways that TB infections can be variable: Populations of cells in different granulomas will evolve differently, individual cells within a granuloma can be different (from the asymmetrical growth) and finally, not all granulomas are equally easy to penetrate.
As mentioned previously, granulomas are little pockets of Mycobacterium tuberculosis surrounded by human immune cells. Drugs must be able to get through this casing of immune cells to reach and kill the bacterial cells. For these reasons, combinations of drugs must be optimized to make sure that treatments have the best chance of killing all TB in a patient, making sure not to leave any behind that could cause the disease to reemerge later.
Combination treatments for TB include four, five or sometimes even more drugs that have to be taken at the same time. However, if you consider all the possible combinations of 4–5 possible drugs and their dosages, the number of options becomes very big, very fast. Work from the Aldridge Lab has shown that considering drug pairs is an effective approach to designing effective combination therapies. Data from in vitro experiments (biological experiments conducted in test tubes rather than live animals) are fed into a machine learning algorithm developed by the lab to predict optimal combinations of four or more drugs.
The idea is that each of these pairs of drugs will be very good at targeting something specific, and then they can be combined to simultaneously attack multiple targets. Aldridge gave the following example.
“You can go camping and somebody gives you a spork, and sure, you can use it to eat soup or mac and cheese, but it does a bad job of both, right? You would much rather have a spoon and a fork. So we find with drug combinations, we need a pair that would do the spoon-like behavior, so that could be, say, synergy in a [fatty] environment … and we need a fork, which might be potency in a sugar-rich environment.”
One pair of drugs will be optimized to target one thing, and another pair of drugs will be optimized to target something else. So instead of trying to find combinations of drugs that are just OK at multiple things, you search for pairs of drugs that are specifically good at doing one specific thing, and you combine them with other drugs that are really good at some other task.
At the end of our conversation, Aldridge left off with some words of advice that are pertinent to share with all current and future researchers who may be reading this article.
“Model organisms are models because they behave weirdly, they grow easily — and that doesn’t make them normal. It just makes them normally studied,” Aldridge said. “And so the more and more different labs are studying the behaviors and lifestyles of actual pathogens, the more we’re learning about the diversity of cell behaviors and what makes them tick is actual pathogens. So I would just encourage people to be bold about what they take on in their research studies — to break away from doing something because it’s the way it’s always been done before.”